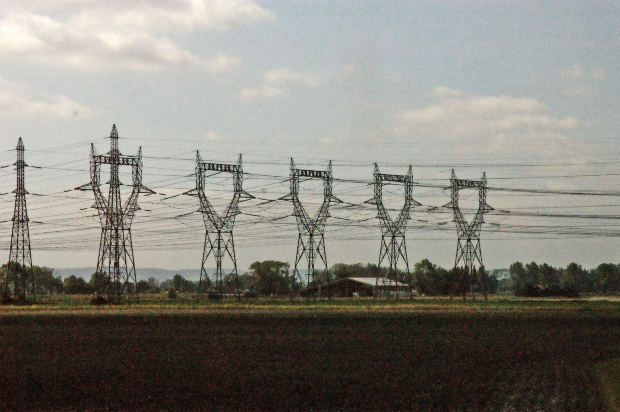
By Chandrashekar Atla,
Load forecasting is of great importance for the management of power systems. Long-term forecasts of the peak electricity demand are needed for capacity planning and maintenance scheduling. Medium-term demand forecasts are required for power system operation and planning. Short-term load forecasts are required for the control and scheduling of power systems. Short-term forecasts are also required by transmission companies when a self-dispatching market or open access market is in operation. It is required for unit commitment, energy transfer scheduling and load dispatch. With the emergence of load management strategies, the short-term load forecast has played a broader role in utility operations. The development of an accurate, fast and robust short-term load forecasting methodology is of importance to both the electric utility and its customers. More generally, error in predicting electricity load has significant cost implications for companies operating in competitive power markets.
Forecasting Methods
Several different methods, techniques and algorithm have been developed to forecast load demands, with the focus on improving the prediction accuracy. The approach using time series analysis (Autoregressive (AR) models) is among the main areas with rich research effort, with specially formulated methods for data in various contexts.The objective of forecasting is to obtain the best possible estimates or forecasts of what will happen in the future based upon the time series model fitted to the historical time series as well as the most recent observations. Time series models constitute an important class of models which can be used for addressing a wide range of challenging problems in the water forecast. A comprehensive data analysis study can be carried out before being used by the time series model.
Univariate models only consider the historical demand data and multivariate model will consider the future weather variables/meteorological variables along with historical demand and weather data. It is well recognized that meteorological variables have a very significant influence on electricity demand. However, in online short-term forecasting systems, multivariate modeling is usually considered impractical. In such systems, the lead times considered are less than a day-ahead, and univariate methods can be sufficient because the meteorological variables tend to change in a smooth fashion, which will be captured in the demand series itself. Indeed, univariate models are often used for prediction up to about four to six hours ahead. For higher lead times, a multivariate model provides good accuracy results.
Forecasting Stages
In general, time series modeling can be categorized into 4 stages:
- Selection of the type of model,
- Identification of the order of the model,
- Estimation of the model parameters, and
- Diagnostic check of the model
The first stage refers to selecting a type of model among the time series models available, i.e. auto regressive models and periodic (to take care seasonality) auto regressive methods based on the data and user specifications. Once a type of model is selected, the next stage is to identify the order of the model. For instance, if autoregressive models were selected in the first stage, then we need to identify the order of autoregressive model say order 1 or order 2 etc. The third stage is to estimate the parameters of the model identified in stage two and some checks are made on the conditions to be met by the estimated parameters. The final stage of the modeling is to make some diagnostic checks to verify how good the model is. The overall time modeling is actually an iterative process with feedback and interaction between each of the above referred stages. The flowchart of the procedure is presented in Figure for quick reference.
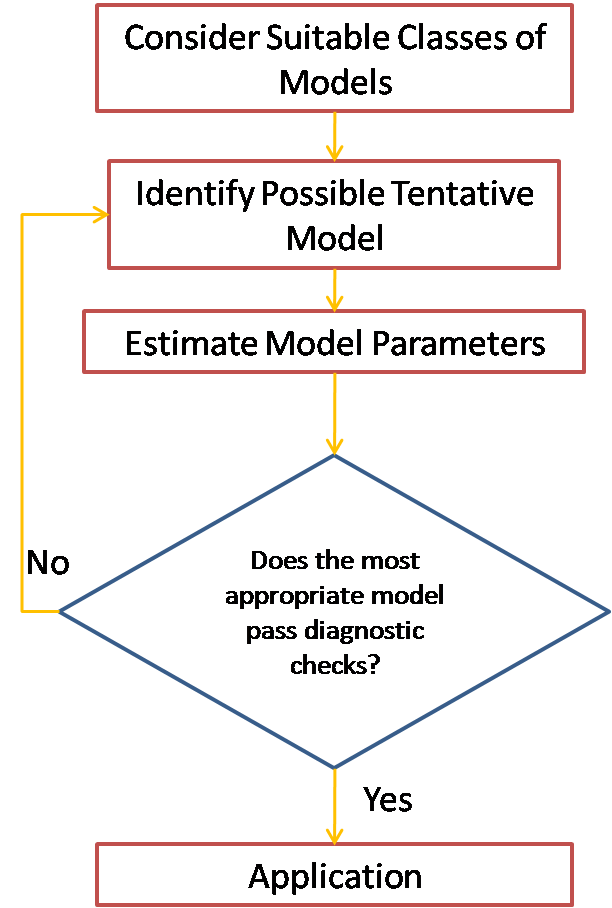
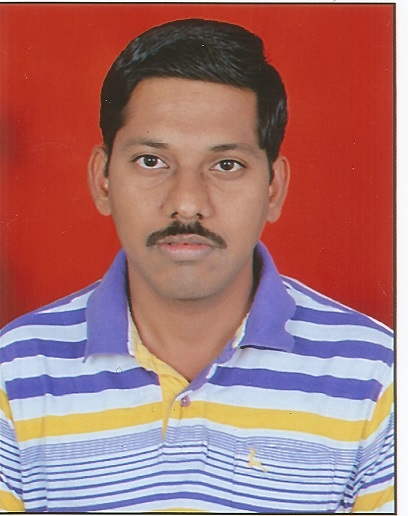
About the author
Chandrashekar is a data scientist at Spider OpsNet. He comes with electrical engineering and mathematics background. He was instrumental in getting long term power forecasting algorithms based on multivariate analysis being built into a product used by our clients in the power industry.